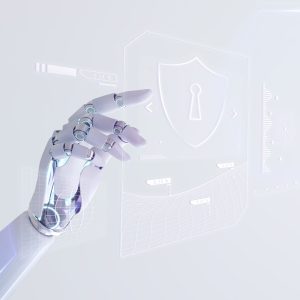
Generative AI in Cyber security risks. As technology advances at an unprecedented pace, so do the challenges in the field of cybersecurity. One such challenge is the rise of generative artificial intelligence (AI).
What is generative AI?
Generative AI is a branch of artificial intelligence that can create new data or content based on existing data or content. Since it learns by itself and generates content by itself, it can pose significant challenges in the field of Cybersecurity. It opens up new avenues for cyber threats and vulnerabilities. In this blog, we will delve into the emerging risks of generative AI in cybersecurity and hence provide valuable insights on how to mitigate these risks effectively.
The Potential Threats of Generative AI in cyber security
- Phishing attacks is more convincing with the help of Generative AI. By mimicking legitimate organizations or individuals, it can create fake login pages or websites that look almost identical to the real ones. This can trick users into giving away sensitive information without realizing it.
- Malware is more dangerous with the use of Generative AI. It can create self-learning code that constantly evolves and adapts to the target system. This means that even if the system has antimalware software, the malware can still evade detection.
- Deepfakes are a growing concern with the use of Generative AI. It can create sophisticated videos, audio, or images that can deceive and mislead viewers. This is used for impersonation, phishing, scamming, or spreading false information. believing it.
- Identity theft is a serious threat that can be made easier with the use of Generative AI. By tricking users into giving away personal information, it can create forged documents that are almost indistinguishable from the real ones. This can lead to serious consequences and damage to one’s reputation.
- Data Poisoning: AI models heavily rely on huge amounts of data for training. If an attacker manages to inject malicious data into the training set, it can undermine the integrity and reliability of the AI model.
- Evasion of IDS/IPS systems: Generative AI is used to develop sophisticated evasion techniques that can bypass security controls, such as intrusion detection systems . These AI-generated attacks can adapt and evolve rapidly, making it challenging for IDS/IPS systems to detect and mitigate them effectively.
Strategies for Mitigating Risks
Robust Data Validation: Implementing strict data validation processes is crucial to ensure the integrity of the training data.
Implement an AI Policy for Your Company: Whether you’re currently utilizing generative AI throughout your company or are considering its advantages, it’s crucial to establish a policy that governs its use. This policy should detail: – The departments and positions that are authorized to use generative models as part of their job responsibilities. – The specific areas of the workflow that can be automated or augmented with generative AI. – The AI can access which internal data and applications and which data and applications are not permitted .
Regularly monitoring and auditing the data used for training generative AI models can help identify and remove any malicious or manipulated data that may compromise the system’s security.
Enhanced Authentication and Authorization: As generative AI can be used to create convincing fake identities; it is vital to strengthen authentication and authorization processes. Implementing multi-factor authentication, can help verify the identity of users and prevent unauthorized access. Hence help in maintaining a secure access process.
Continuous Monitoring and Threat Intelligence: Additionally Investing in advanced threat intelligence systems and continuous monitoring tools can help organizations stay one step ahead of potential AI-generated attacks. These systems can detect anomalies, identify emerging threats, and proactively respond to potential security breaches.
Investing in advanced cyber security technologies like the following ones that are designed with AI and other modern attack surfaces in mind:
- Identity and access management.
- Data encryption and data security tools.
- Cloud security posture management (CSPM).
- Penetration testing.
- Extended detection and response (XDR).
- Data loss prevention (DLP).
Ethical Considerations:
Organizations must adopt ethical frameworks and guidelines for the development and deployment of generative AI systems. It is crucial to ensure transparency, accountability, and responsible usage of AI technologies . This is essential to prevent misuse and minimize the potential generative AI risks.
Conclusion: Generative AI undoubtedly holds immense promise for various industries, but it also introduces new risks and challenges in cybersecurity. By understanding these risks and implementing effective mitigation strategies, organizations can safeguard themselves against potential threats.
By raising awareness and taking proactive measures, we can harness the power of generative AI while minimizing its potential risks.